More info
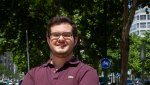
Miguel Costa
PhD Candidate
Filipe Moura
Supervisor
Manuel Marques
Co-supervisor
Carlos Lima Azevedo (DTU)
Co-supervisor
Keywords
Cycling Safety · Urban Environment · Objective and Subjective Safety · Machine Learning in Transportation · Risk Perception in Cycling
Funding
This research is supported by the Portuguese Foundation for Science and Technology (FCT), through the Ph.D. grant PD/BD/142948/2018.